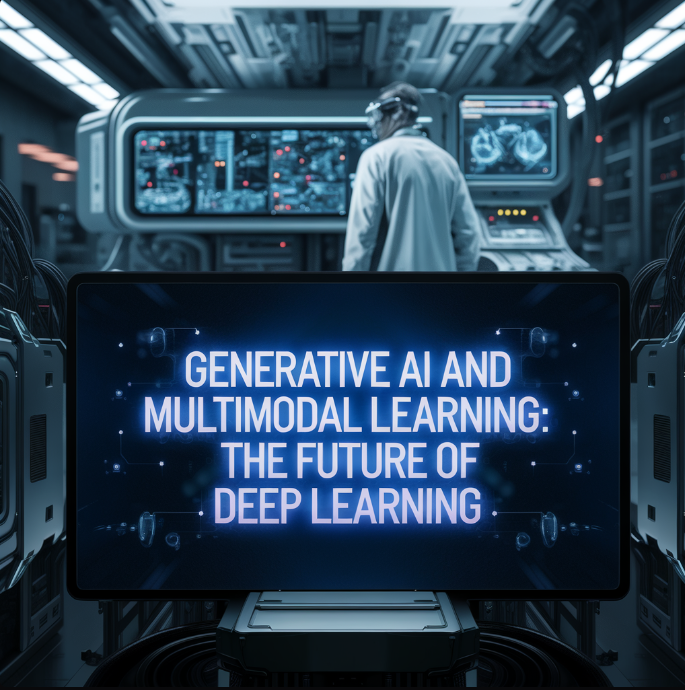
Generative AI and Multimodal Learning: The Future of Deep Learning
The last few years have witnessed the rise of Generative AI and Multimodal Learning as two of the most promising areas in deep learning. Both these technologies have the ability to completely change the way artificial intelligence (AI) works which in turn allows machines to generate content that is almost as real as that created by humans and get information from various sources at the same time. As we advance through 2024, both of these areas are moving fast; they will create a world in which AI can generate, understand, and merge different types of media like text, images, video, and more.
In this article, we will examine the ideas behind generative AI and multimodal learning, their uses, and the potential future impact in a wide range of industries such as entertainment, and medical.
What is Generative AI?
Generative AI means systems that have the capability to produce original content by themselves. This content could be in the form of text, images, audio, video, or even more complex content such as code or 3D objects. Generative AI models, which are encoded using a certain approach called deep learning, are supplied with several datasets from which information is reformed and new features or data structures are created that closely imitate the original ones. Among the known generative models are Generative Adversarial Networks (GANs) and Variational Autoencoders (VAEs).
How Generative AI Works:
Generative AI is fundamentally based on two elements:
- A generator that produces fresh data samples.
- A discriminator that determines if the data is produced or authentic.
The generator’s outputs should be realistic enough to “fool” the discriminator into believing they are real. This dynamic eventually produces very realistic synthetic data that can be hard to tell apart from real data.
Multimodal Learning: Integrating Diverse Inputs:
The process by which AI models combine several types of data to complete a job is known as multimodal learning. In the past, machine learning models were trained on a particular type of input, like images in computer vision or text in natural language processing (NLP). Multimodal learning, on the other hand, attempts to overcome these silos by developing models that can comprehend and learn from a variety of data formats, such as:
- Text
- Images
- Audio
- Video
- Sensory data (e.g., touch, temperature)
By combining these inputs, multimodal models create a richer, more holistic understanding of information, allowing for more robust and versatile AI systems.
How Multimodal Learning Works:
Neural architectures that can process many data sources simultaneously are frequently used in multimodal models. Usually, this includes:
- Separate feature extractors (such as a transformer for text and a convolutional neural network for images) for every kind of data.
- A fusion layer that creates a shared representation by combining the extracted features.
Combining the features enables the model to make decisions based on all of the information it has been given, which improves performance on challenging tasks like question answering, content creation, and even human-AI interaction.
Key Advances in Generative AI and Multimodal Learning:
- Transformers and Large Language Models:
Generative AI has advanced to new heights thanks to large language models (LLMs) like GPT-4 and GPT-5. The architecture behind these models, Transformers, enables the production of high-quality, contextually appropriate text while managing large datasets.
As LLMs become more sophisticated, they can do more than just text. In order to create systems that can produce not only text but also comparable images or videos—like AI-generated movies or audio captions for videos—multimodal transformers that combine images, video, and audio into a single model are currently being developed.
2. DALL·E and Image Synthesis:
DALL·E, an AI model created by OpenAI that can create visuals from textual descriptions, is among the most well-known uses of generative AI. DALL·E allows users to enter phrases like “a sunset over a mountain range” and the AI will produce a photorealistic image. Models that can produce increasingly more sophisticated and high-resolution visuals, including 3D objects or animations, in response to challenging requests are starting to appear in 2024.
3. CLIP and Cross-Modal Learning:
One example of how multimodal learning is changing AI’s capacity to comprehend and relate many data kinds is OpenAI’s CLIP. Because CLIP has been trained to acquire combined representations of text and images, it can carry out tasks like text-based image search and image captioning, in which the AI uses natural language to explain the contents of an image.
By combining these features, CLIP is a prime example of how multimodal AI models may “understand” the connections between words and images, creating new opportunities for search engines, content production, and even self-governing systems.
Applications of Generative AI and Multimodal Learning:
- Content Creation and Entertainment:
The entertainment sector is already utilising generative AI for jobs like digital art production, video game development, and scriptwriting. While generative audio models can create music or voiceovers for virtual characters, artificial intelligence (AI) models such as DeepMind’s AlphaStar are being utilised to build complete virtual worlds.
A new era of creativity is being ushered in with the emergence of further tools in 2024 that let even non-experts to use generative AI to produce professional-caliber material.
2. Healthcare:
Multimodal learning has the ability to completely transform treatment planning and diagnosis in the medical field. Multimodal AI models can offer more precise diagnoses and individualised treatment suggestions by combining data from patient records, medical imaging (such as MRIs), and even genetic information. In order to train other AI systems, generative models are also being utilised to create synthetic medical data or to mimic the effects of novel medications.
3. Human-AI Interaction:
AI is getting better at communicating with people in natural ways as multimodal models are developed. These days, chatbots, such as OpenAI’s ChatGPT, can comprehend visual inputs, reply to voice commands, and provide text or audio responses. These developments open the door to increasingly sophisticated and immersive virtual assistants that are capable of performing a variety of duties, such as virtual tutoring and customer service.
4. Autonomous Systems:
The progress of robotics and driverless cars depends on multimodal learning. Multimodal AI can make judgements in real time in dynamic contexts by analysing visual, aural, and sensor data simultaneously, enhancing the safety and dependability of drones and self-driving cars.
The Future of Generative AI and Multimodal Learning:
The potential of multimodal learning and generative artificial intelligence is enormous as we look to the future. As model designs and training methods advance, we can anticipate that these technologies will become even more prevalent in fields like entertainment and healthcare. Over the next few years, we should expect to see:
- . AI models that are more individualised and capable of producing original content based on user choices.
- In real-time applications, where generative AI can help with real-time decision-making or produce realistic simulations.
- Multimodal AI systems that can comprehend human input from many sources (speech, gestures, etc.) and react in ways that are appropriate for the situation will improve AI-human collaboration.
Multimodal learning and generative AI are coming together to pave the way for a time when AI will be able to think, create, and interact more like humans, revolutionising the way we work, play, and live.
Conclusion:
A new phase of deep learning is represented by generative AI and multimodal learning, in which AI systems can comprehend and integrate many kinds of information in addition to producing content. By making AI more creative, clever, and able to comprehend the world as humans do, these technologies are expanding the realm of what artificial intelligence is capable of. We may anticipate even more developments in these areas as they develop further, which will alter how humans and AI interact with one another.
Now is the ideal moment for developers, data scientists, and AI enthusiasts to explore the exciting fields of multimodal learning and generative AI. With the correct resources and information, the options are virtually limitless.